28% of Taiwan’s Electronics and Information Manufacturing Industry Implements AI
The Market Intelligence & Consulting Institute (MIC) recently released the survey results on the adoption of AI in Taiwan’s electronics and information manufacturing industry. The survey reveals that 28% of enterprises in the industry have implemented AI, with another 46% currently in the planning stage. Looking at sub-industries, AI application development is more mature in the PCB, optoelectronics materials, and components industries, while the development in other electronic components, computers, and peripheral equipment industries is relatively slower.
The survey points out that large enterprises are ahead of small and medium-sized enterprises (SMEs) in AI deployment progress. However, SMEs are accelerating their efforts, with the compound annual growth rate (CAGR) of AI investments expected to reach 26% from 2024 to 2026. MIC industry analyst Zhang Jiafu stated that the primary goals of AI adoption by manufacturers are to improve performance and reduce costs. The key metrics they focus on include yield, production capacity, time-to-market, and cost.
AI Investment Continues to Grow, with Hardware Spending Having the Highest Share
The survey results show that enterprises that have already implemented AI plan to invest an average of NT$2.09 million in 2024, with the amount expected to rise to NT$2.36 million in 2025 and NT$2.61 million in 2026, reflecting an 11.5% CAGR over the three years. Around 40% of businesses are continuously increasing their AI investments, with 46% expected to increase their budget in 2025 and 39% in 2026.
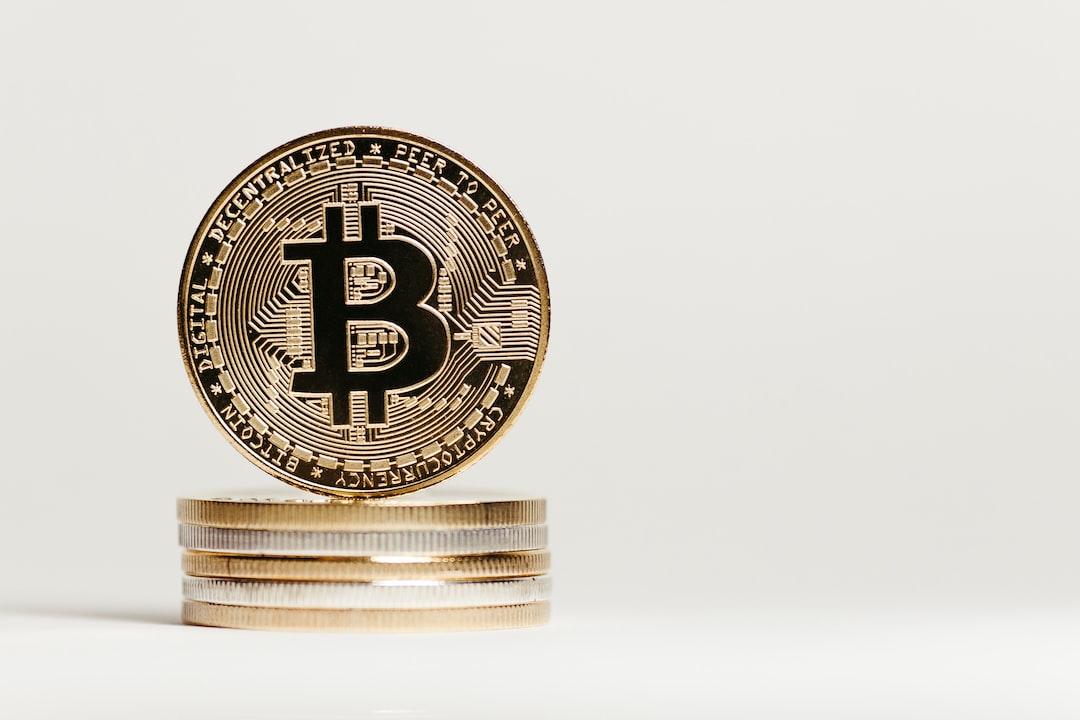
AI investment forecast for enterprises that have implemented AI.
Graph / Future Business Creation (Data from MIC)
In terms of resource allocation, in 2025, the manufacturing industry’s hardware spending will account for the largest share of AI investments, reaching 46%, followed by software (42%), and services (12%), which has the lowest share. This indicates that Taiwan’s automation industry has strong competitiveness in the hardware field, and future business opportunities are promising.
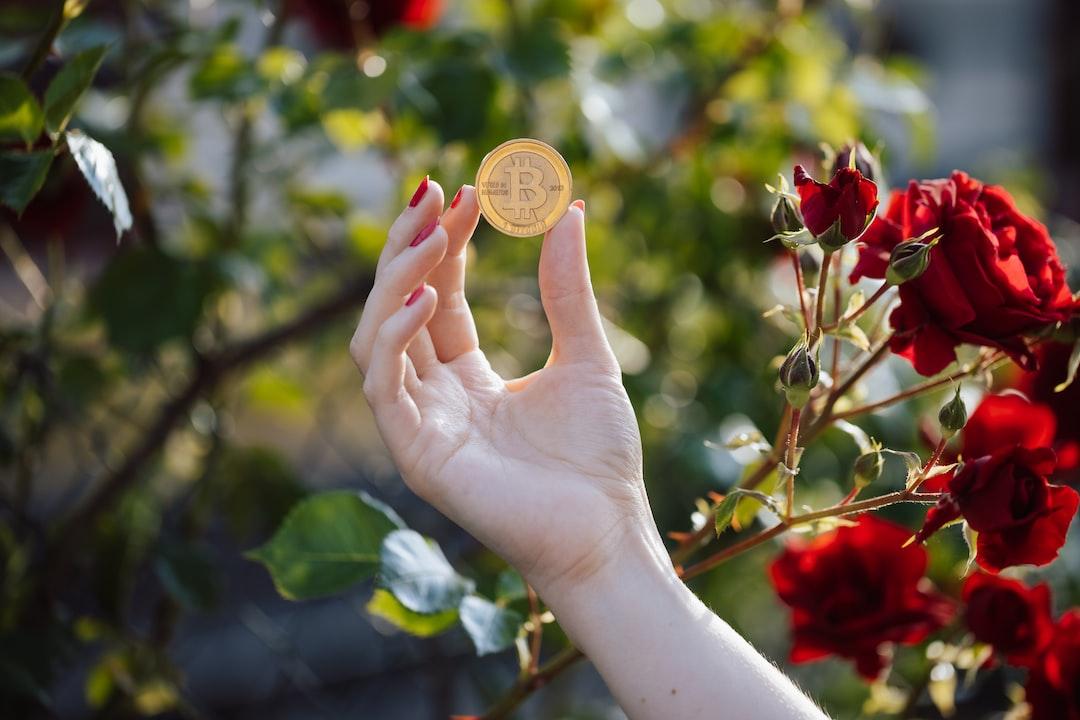
Resource allocation for AI investments in the manufacturing industry in 2025.
Graph / Future Business Creation (Data from MIC)
Observation 1: Discriminative AI is the Mainstream, Generative AI’s Potential Yet to Be Explored
The MIC points out that in terms of AI technology investment, the manufacturing industry still mainly focuses on discriminative AI, with a budget allocation ratio of 73% in 2025, far surpassing generative AI at 27%. In 2026, the investment share of generative AI is expected to slightly increase to 29%. When focusing on AI applications in manufacturing units, the number of companies using discriminative AI is 1.6 times greater than those using generative AI.
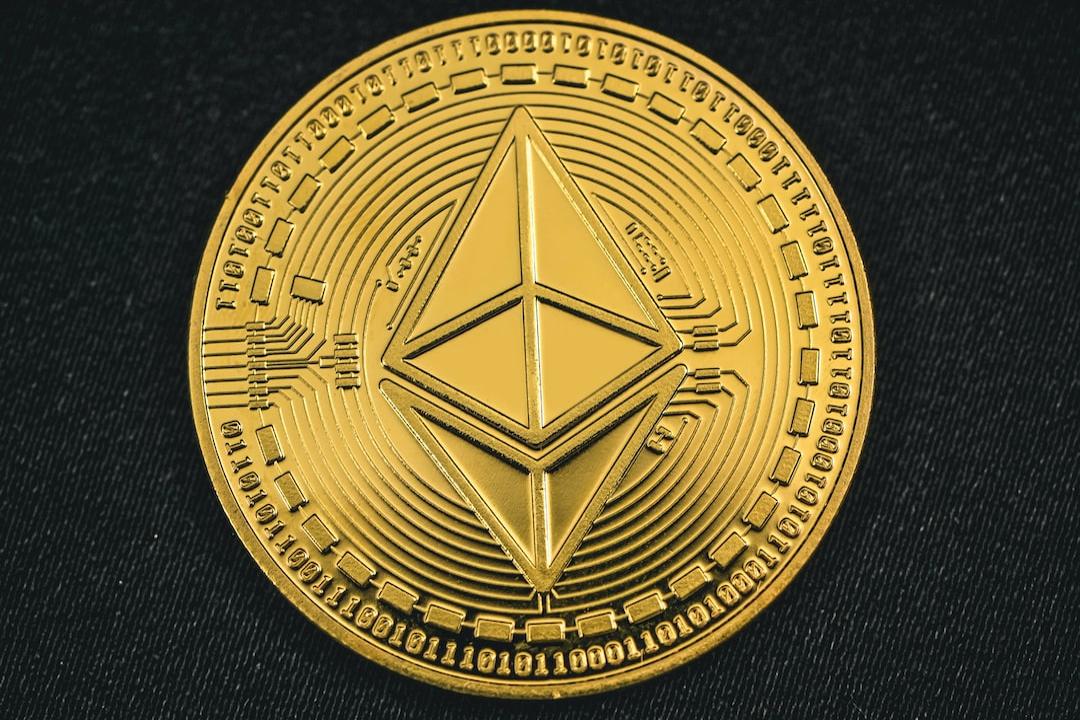
Budget allocation for discriminative and generative AI in manufacturing in 2025.
Graph / Future Business Creation (Data from MIC)
Although generative AI applications currently focus mainly on “product development report generation” and show relatively low satisfaction, with the development of AI agents and human-machine collaboration technologies, generative AI is expected to expand into more manufacturing and production processes in the future. Zhang Jiafu suggests that solution providers should continue to develop related applications to seize emerging market opportunities.
Observation 2: Quality Control and Production Are the Mainstream AI Applications
Among the top ten AI applications, half are related to manufacturing production, with the top three being defect detection, defect image labeling, and production process improvement. In the future, the demand for AI in manufacturing and production departments will remain the highest, followed by product R&D and product quality inspection departments, indicating that these departments will further close the gap in terms of intelligence compared to other departments.
The MIC survey indicates that the top ten AI applications are: defect detection, defect image labeling, production process improvement, product development reports, root cause analysis of defects, production scheduling planning, detection of design flaws, workplace accident analysis, process parameter optimization, and production problem root cause analysis.
Observation 3: IT Department as the Core Driver of AI
Among the enterprises that have implemented AI, the IT department has the fastest AI development progress, with a 60% implementation rate, indicating that the IT department is generally the driving force behind the company’s digital transformation. The next fastest departments are manufacturing production and product quality inspection, which are also the areas where AI applications are most concentrated.
AI Implementation Satisfaction and Challenges: Data is the Core Issue
The survey shows varying degrees of satisfaction with the outcomes of AI implementation, with the most significant improvements including increased revenue, reduced labor shortage pressure, and lower costs. However, satisfaction with “improving problem predictability” is relatively low. Zhang Jiafu analyzed that the unsatisfactory predictive ability of AI might be influenced by market supply-demand changes, political and economic environments, and the company’s own data readiness.
The MIC points out that data issues remain the biggest challenge for AI development in the manufacturing industry, with 80% of enterprises that have implemented AI facing data-related difficulties. Large enterprises, in particular, face more complicated data management issues due to their complex organizational structure. Furthermore, enterprises that are still in the planning stages of AI implementation mainly face high costs and difficulties in evaluating benefits.
Industry analyst Zhang Jiafu states that data readiness can only be assessed through actual implementation. Insufficient data results in poor model performance, while excessive data that is not properly governed can also prevent AI from reaching its full potential. Enterprises should start with the end in mind, first confirming the AI application scenarios, then planning the required data to ensure data volume, quality, and governance are in place, thus improving the accuracy and effectiveness of AI applications.
This article is reprinted with permission from: Future Business